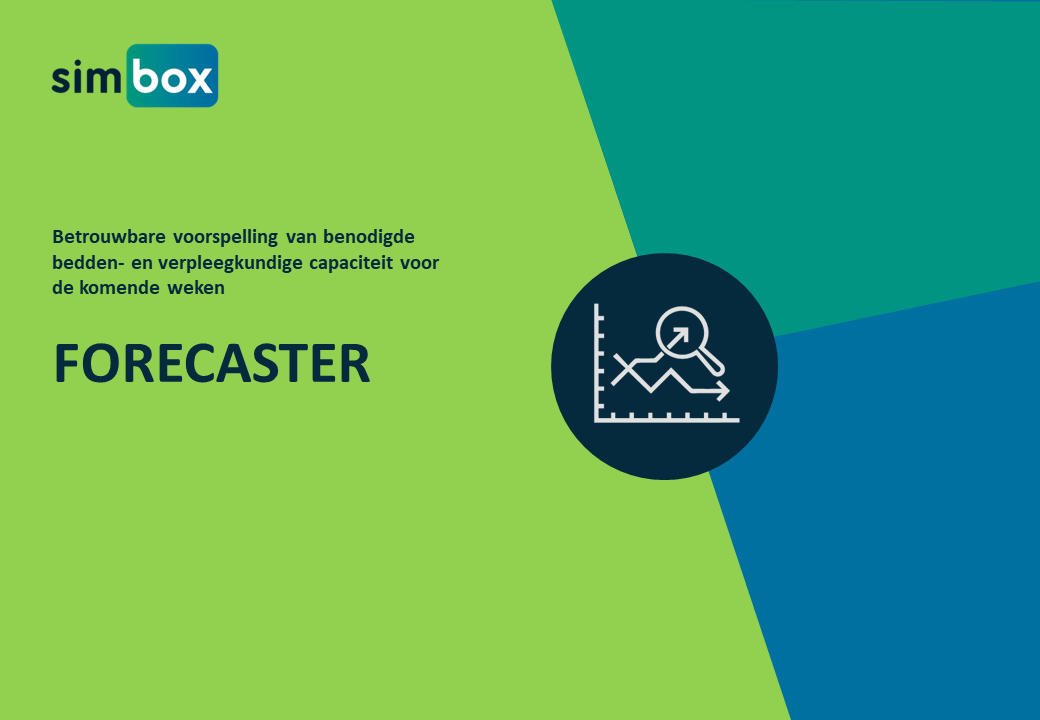
To anticipate is to rule
One thing is certain in hospitals, they have to deal with a lot of variation in the demand for capacity. Every day can be different. With that much uncertainty in the exact demand, it is difficult to make the right decisions. But imagine that this uncertainty suddenly no longer turns out to be uncertain, but that it is very easy to predict where that variation comes from. In that case, the variation will no longer happen to you, but you will see the busy and quiet moments coming in advance and you can react in time.
This is precisely the purpose of forecasting. A forecasting algorithm tries to detect patterns in the historical data of the demand for capacity in all sorts of ways. In the emergency room, for example, it is much quieter at night than during the day. These learned patterns can then be projected into the future.
So the principle of forecasting actually sounds very simple. However, there are many different ways how to predict, and in a hospital there are many aspects to consider. If we have to outline it, it comes down to the following.
The demand for beds is determined by the combination of inflow and throughput of patients. If the inflow is low and the length of stay is very high, the demand for beds may be the same as in the case where the inflow is high and the length of stay very short.
Therefore, a bed demand forecast can be split into two separate forecast models. One for the inflow, and a second for the throughput. In a hospital, many patient groups can be defined, each behaving in their own way in terms of inflow and throughput. These groups form the basis for the forecast.
For each patient group, it is necessary to make a good prediction of the inflow. Part of this is already known at the moment of prediction, namely the patients who are already present and the patients who are already scheduled. However, emergency patients and patients yet to be scheduled are not known in advance. These are therefore predicted. For this, the model is specifically tuned to the patient group. In other words, the best prediction model is selected for each patient group. And this is done fully automatically.
Once the inflow is known, it is time to make a prediction of the throughput. We know from experience that a patient can flow through the hospital in many different ways. And these flows are time dependent on the one hand and path dependent on the other. For example, no patient will be transferred at the weekend to a department that is closed during the weekend. But also, an admission with a long length of stay is more likely to be planned for a Monday than a Friday in a ward that closes at the weekend. The partial path that a patient has already taken also matters for the continuation of the path and the expected length of stay. For example, a patient arriving at a nursing ward via the ED is likely to spend longer in that same nursing ward than an elective admission.
By combining both prediction models, a prediction for the coming weeks becomes possible. Even though we have tried everything to predict as accurately as possible, we know for sure that this prediction will never be exact. Therefore, in addition to the expected demand for capacity, it is important to show a confidence interval, in which the actual demand will be with a certainty of 95%. Or any other desired percentage.
If we compare the forecast with the available bed capacity, it quickly becomes clear at which moments there will be bottlenecks, but also at which moments there is enough capacity left to plan extra admissions. By providing this insight, bed capacity can be utilised much better.
Of course, there may be a bed available, but to provide care, staff is also needed. It is therefore necessary to translate the patient presence into the nursing staff required. This is done by means of norms, whereby the norm tells, as it were, how many nurses are needed per patient present. These norms should depend on the type of department, but also on the shift. For example, less care will be provided at night compared to during the day, which means that relatively fewer nurses are needed.
The staffing requirement can also be set off against the actual planned formation. In this way, the forecast not only provides insight into the bed capacity, but also into whether the staff schedule is properly attuned to what is required.
This makes the forecaster indispensable in hospitals. It detects explainable and ordered patterns in the large amount of variation, which for many people seems inexplicable and completely random, and displays this in such a way as to enable operational control.